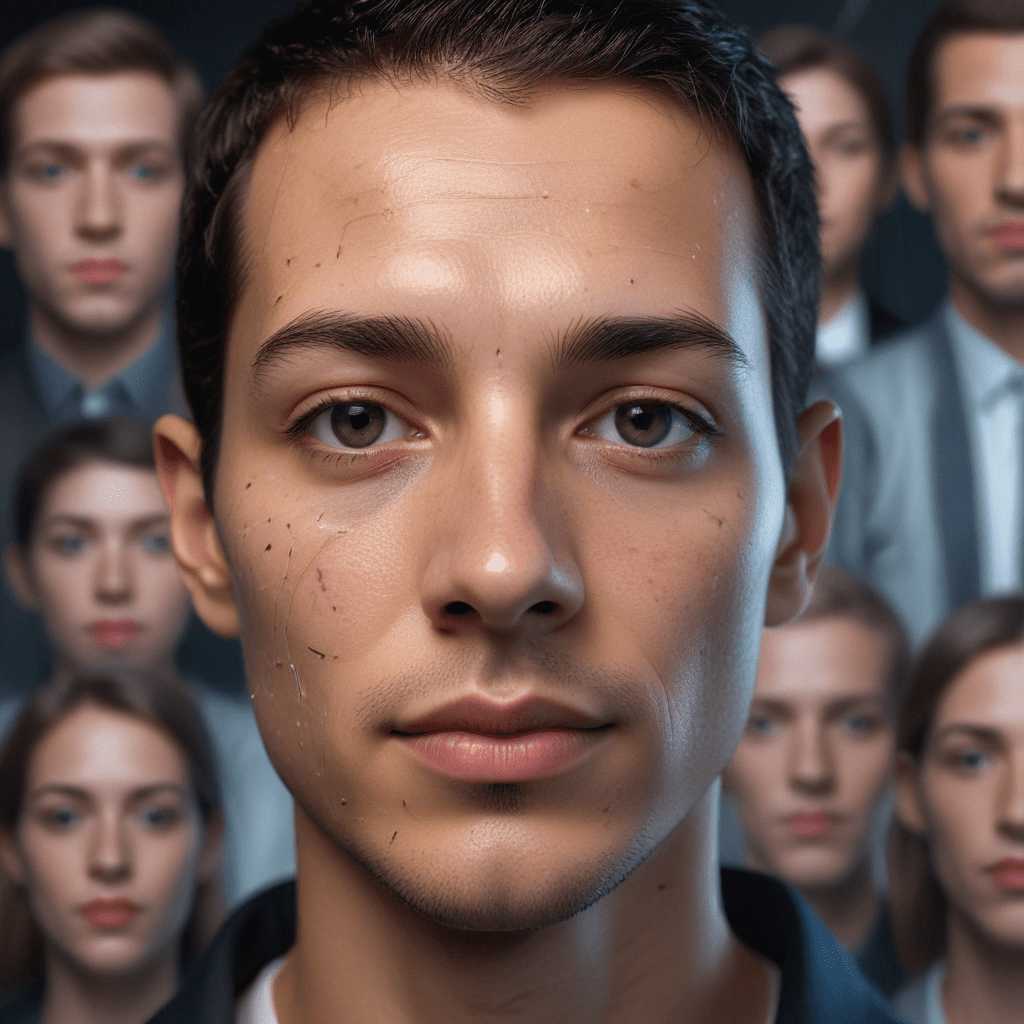
Introduction
Facial recognition technology has become an increasingly prevalent tool in our society, used for everything from security and surveillance to unlocking our phones. However, this technology has also raised concerns about potential bias, as studies have shown that facial recognition algorithms can be less accurate in identifying people of color, women, and other marginalized groups.
Understanding Bias in Facial Recognition
Bias in facial recognition algorithms refers to systematic errors that lead to incorrect or unfair identification results for certain demographic groups. These errors can occur due to a variety of factors, including:
- Data bias: The training data used to develop facial recognition algorithms may be skewed toward certain demographic groups, leading the algorithm to perform better on those groups.
- Algorithmic bias: The algorithm itself may contain biases that lead to unfair results for certain demographic groups. For example, an algorithm may be trained on a dataset that contains more images of white male faces than faces of other demographic groups, leading it to be more accurate at identifying white male faces.
- Human bias: Facial recognition systems are often designed and deployed by humans, and human biases can be introduced into the system at any stage of the process, from data collection to algorithm design to deployment.
Causes of Bias
The causes of bias in facial recognition algorithms are complex and multifaceted. Some of the most common causes include:
- Lack of diversity in training data: Facial recognition algorithms are trained on large datasets of images, and if these datasets lack diversity in terms of race, gender, and other demographic factors, the algorithm may learn to make biased predictions.
- Algorithmic design choices: The design of a facial recognition algorithm can also lead to bias. For example, algorithms that rely on certain facial features, such as the shape of the eyes or nose, may be more likely to misidentify people from certain demographic groups.
- Human bias: Human bias can be introduced into a facial recognition system at any stage of the process, from data collection to algorithm design to deployment. For example, a human may make a biased decision to use a facial recognition system in a way that disproportionately affects certain demographic groups.
Consequences of Bias
Bias in facial recognition algorithms can have significant consequences for individuals and society as a whole. Some of the potential consequences of bias include:
- False positives and false negatives: Biased algorithms may lead to false positives, in which the algorithm incorrectly identifies someone as a match when they are not, or false negatives, in which the algorithm fails to identify someone as a match when they are.
- Discrimination and profiling: Biased algorithms can be used to discriminate against certain demographic groups. For example, a biased algorithm may be used to target people of color for increased surveillance or to deny them access to certain services.
- Loss of trust: Public trust in facial recognition technology is essential for its successful deployment. However, if people believe that the technology is biased, they are less likely to trust it and use it.
6. Technical Solutions
Technical solutions can be employed to detect and mitigate bias in facial recognition algorithms. These solutions include:
- Data augmentation: Adding synthetic data to the training dataset to increase the diversity of the data.
- Algorithmic fairness: Developing new algorithms that are less likely to be biased.
- Bias mitigation techniques: Applying techniques to the algorithm or data to reduce bias, such as preprocessing the data to remove biased features or using fairness-aware learning algorithms.
7. Policy and Regulation
Policy and regulation can also play a role in addressing bias in facial recognition algorithms. Governments can implement regulations that require facial recognition systems to be tested for bias and to meet certain accuracy standards. They can also create laws that prohibit the use of facial recognition systems for certain purposes, such as surveillance or discrimination.
8. Social and Ethical Responsibility
Companies and organizations that develop and deploy facial recognition technology have a social and ethical responsibility to ensure that their products are fair and unbiased. This includes implementing the technical solutions and policy recommendations described above, as well as conducting regular audits to assess the fairness of their systems.
9. Future Challenges
There are still a number of challenges that need to be addressed in order to fully address bias in facial recognition algorithms. These challenges include:
- Measuring bias: Developing effective and reliable methods for measuring bias in facial recognition algorithms.
- Mitigating bias in real-world applications: Bias in facial recognition algorithms can be more difficult to detect and mitigate in real-world applications, where the data is often noisy and the environment is uncontrolled.
- Addressing the root causes of bias: Bias in facial recognition algorithms is often a reflection of deeper societal biases. Addressing these root causes is essential for creating a more just and equitable society.
10. Conclusion
Bias in facial recognition algorithms is a serious problem that can have significant consequences for individuals and society as a whole. It is essential that we address this problem through a combination of technical solutions, policy and regulation, and social and ethical responsibility. By working together, we can create a future in which facial recognition technology is used fairly and equitably for the benefit of all.
FAQ
Q: What is bias in facial recognition algorithms?
A: Bias in facial recognition algorithms refers to systematic errors that lead to incorrect or unfair identification results for certain demographic groups.
Q: What are the causes of bias in facial recognition algorithms?
A: The causes of bias in facial recognition algorithms are complex and multifaceted, but some of the most common causes include lack of diversity in training data, algorithmic design choices, and human bias.
Q: What are the consequences of bias in facial recognition algorithms?
A: Bias in facial recognition algorithms can have significant consequences for individuals and society as a whole, including false positives and false negatives, discrimination and profiling, and loss of trust.
Q: What can be done to address bias in facial recognition algorithms?
A: Bias in facial recognition algorithms can be addressed through a combination of technical solutions, policy and regulation, and social and ethical responsibility.